Digital phase contrast image analysis today combines traditional microscopy with AI-powered computational tools to examine living cells without staining. You’ll find it uses specialized hardware (phase objectives, digital cameras) and advanced software featuring machine learning algorithms that reduce artifacts and automate cell counting. Modern systems achieve remarkable accuracy with deep learning, particularly CNNs, while providing quantitative metrics on cell morphology and behavior. The technology continues to evolve as researchers overcome challenges with cell density and signal-to-noise ratios.
The Evolution of Digital Phase Contrast Microscopy
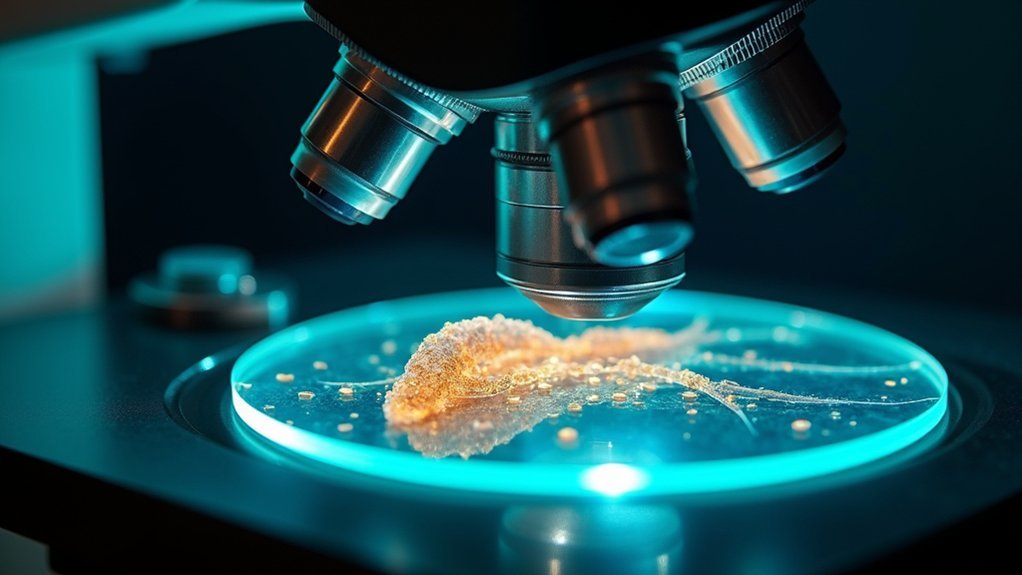
While Frits Zernike pioneered phase contrast microscopy in the 1930s, the technique has undergone remarkable transformation with the advent of digital technologies.
You’ll find today’s digital phase contrast microscopy offers unprecedented capabilities for observing living cells without staining them.
The integration of advanced digital imaging has revolutionized how researchers capture and analyze cell images. You can now monitor cellular processes in real-time with greater clarity and precision.
Modern systems incorporate sophisticated restoration algorithms that effectively minimize traditional artifacts like halos and shade-off effects.
Perhaps most impressively, artificial intelligence has entered the field, with convolutional neural networks automating cell counting and analysis.
These systems achieve impressive accuracy with MSE values as low as 4,335.99 for certain cell lines, making your research more efficient and reliable.
Core Principles Behind Phase Contrast Imaging Technology
Understanding the fundamental mechanisms of phase contrast imaging reveals how this revolutionary technology makes the invisible visible. When you observe specimens under phase contrast microscopy, you’re witnessing a clever optical trick developed by Frits Zernike in the 1930s.
The system uses an annular diaphragm and quarter-wave phase plate to transform phase shifts (caused by varying optical densities in different cell regions) into amplitude changes that your eye can detect. Unlike traditional methods requiring stains that often kill specimens, this technique creates high-contrast images of living cells without disrupting their natural processes.
Though artifacts like halos can complicate interpretation, modern digital analysis techniques, including machine learning algorithms, help overcome these limitations. This enables more precise cell counting and structural analysis in real-time biological research.
Hardware Requirements for Digital Phase Contrast Analysis
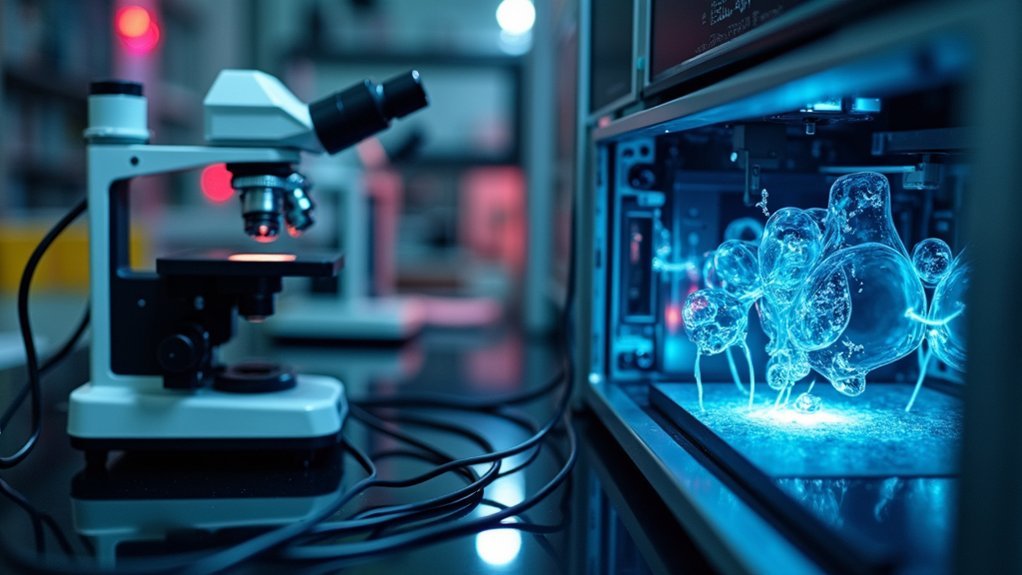
Three essential components form the backbone of any digital phase contrast imaging system.
First, you’ll need a phase contrast microscope equipped with specialized objectives and a condenser containing an annular aperture to modify light paths for transparent specimens.
Second, your setup requires a quality light source—either halogen or LED—to properly illuminate samples and achieve maximum contrast.
Finally, don’t overlook digital acquisition and processing hardware. You’ll need a high-resolution digital camera to capture detailed images, as the quality directly impacts cell detection accuracy.
Your computer must have sufficient processing power and memory to run analysis software and handle large datasets generated during imaging.
These hardware requirements guarantee reliable digital phase contrast analysis for your research or diagnostic applications.
Software Solutions for Enhanced Phase Contrast Processing
Modern software solutions have revolutionized phase contrast image analysis by tackling its inherent limitations. These platforms incorporate advanced image restoration algorithms that effectively reduce halo and shade-off artifacts, considerably improving image quality for your research.
You’ll find many digital analysis tools now leverage machine learning, particularly CNNs, to automate cell counting and feature recognition without requiring cell staining—preserving cellular integrity during high content screening of large datasets.
The software typically offers customizable parameters for image segmentation and quantification, allowing you to tailor analysis to specific specimen types or experimental conditions.
Additionally, integration with other imaging modalities like fluorescence microscopy enhances functionality, providing you with extensive insights into dynamic biological processes through a single analytical platform.
Computational Algorithms in Modern Phase Contrast Analysis
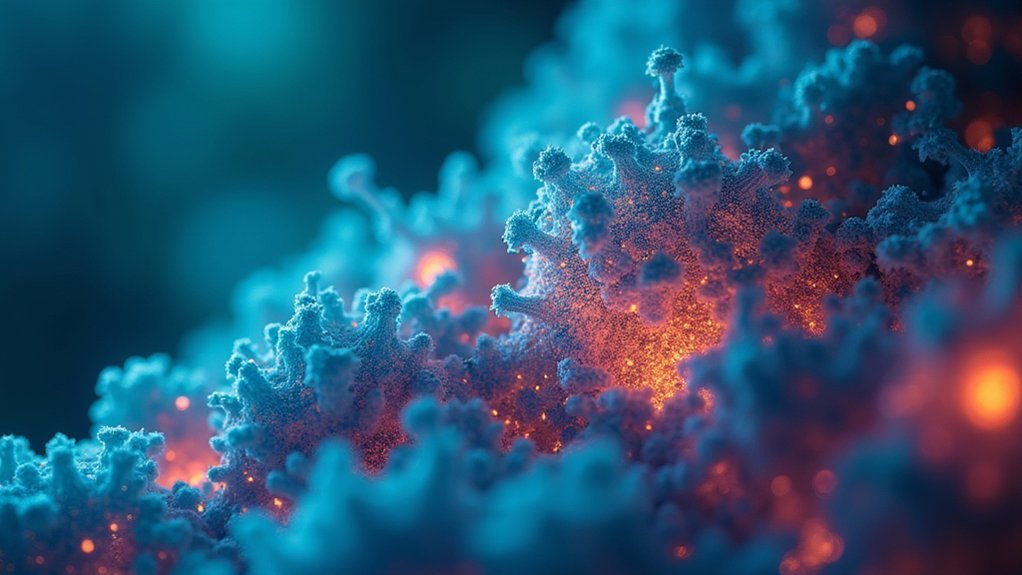
You’ll find that deep learning applications have revolutionized phase contrast analysis through CNNs that efficiently extract detailed cellular information while minimizing common artifacts.
These neural networks excel at feature extraction techniques, enabling automated identification of cell boundaries and morphological characteristics with markedly reduced MSE values compared to traditional methods.
Regularized cost functions further enhance these capabilities, allowing you to track dynamic cellular processes in real-time with unprecedented accuracy and detail preservation.
Deep Learning Applications
Recent advancements in computational algorithms have revolutionized phase contrast image analysis, with deep learning emerging as a powerful tool for cell quantification and characterization. You’ll find Convolutional Neural Networks (CNNs) particularly effective, achieving impressive accuracy for cell counting in phase contrast light microscopy with MSE values as low as 4,335.99 for A549 cells.
Cell Line | Correlation (R) | MSE | Challenges |
---|---|---|---|
A549 | 0.953 | 4,335.99 | Limited training data |
Huh7 | 0.821 | Higher | Complex morphologies |
3T3 | Lower | Highest | Poor signal-to-noise ratio |
Data augmentation dramatically expands your training datasets from 167 to 2,673 images, improving model performance across cell lines. While A549 and Huh7 cells show strong predictive results, challenges remain with 3T3 cells due to density and image quality issues.
Feature Extraction Techniques
Feature extraction stands at the core of successful computational analysis in phase contrast microscopy. When you’re processing these images, advanced algorithms help you extract meaningful cellular characteristics while minimizing artifacts like halo and shade-off that often obscure true morphology.
Modern segmentation methods leverage machine learning and CNNs to identify cell boundaries with unprecedented accuracy. You’ll find these algorithms particularly effective because they’re designed to recognize complex patterns that traditional techniques might miss.
By implementing data augmentation, you can expand your training datasets, reducing MSE in cell counting applications.
The integration of restoration algorithms within your analysis pipeline considerably enhances image quality before feature extraction begins. This thorough approach guarantees robust performance when you’re conducting automated cell analysis, even with challenging samples.
Applications of Digital Phase Contrast in Biomedical Research
While traditional microscopy techniques often require cell staining that can disrupt cellular processes, digital phase contrast microscopy has revolutionized biomedical research by enabling real-time visualization of living cells without chemical interference.
You’ll find this technology particularly valuable for monitoring dynamic cellular behaviors in A549 and Huh7 cell lines.
Digital phase contrast excels in developmental biology and pathology applications where preserving natural cell behavior is essential. The integration of CNNs has dramatically improved cell tracking capabilities, with MSE values as low as 4,335.99 for A549 cells.
Modern segmentation algorithms effectively overcome common artifacts like halo and shade-off effects, making automated analysis more reliable.
You can now quantify and analyze cellular morphology with unprecedented accuracy, providing vital insights into disease mechanisms that were previously difficult to observe in real-time conditions.
Quantitative Analysis Techniques for Cell Morphology
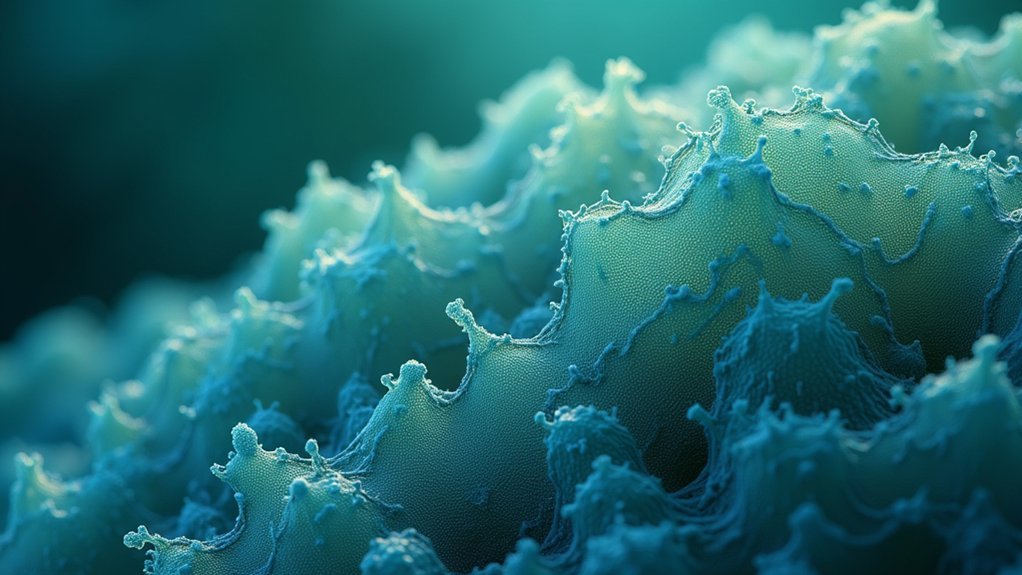
Through sophisticated algorithmic processing, digital phase contrast imaging transforms qualitative visual data into precise numerical measurements of cellular structures.
You’ll find that quantitative analysis techniques now leverage advanced segmentation algorithms and restoration methods to enhance image quality by reducing halo and shade-off artifacts.
- Machine learning models, especially CNNs, automate cell feature quantification with lower MSE values
- Data augmentation through rotation and scaling improves model robustness
- Segmentation algorithms precisely delineate cell boundaries for accurate measurements
- Cell morphology analysis reveals critical biological characteristics without staining
- Quantitative measurements include cell size, shape, and density metrics
These approaches enable you to extract meaningful data about cell morphology from phase contrast images, supporting research in developmental biology and pathology with unprecedented precision and efficiency.
Challenges and Limitations in Digital Phase Contrast Imaging
While digital phase contrast imaging offers powerful visualization capabilities, you’ll encounter fundamental trade-offs between resolution and sensitivity that impact cellular detail detection.
The computational demands for processing these complex optical datasets require significant resources, particularly when implementing real-time analysis or processing large experimental datasets.
Current reconstruction algorithms still struggle with artifacts like halos and shade-off effects, especially in challenging conditions such as high cell density environments or when imaging 3T3 cell lines with inherently low signal-to-noise ratios.
Resolution Versus Sensitivity
Despite significant advances in digital phase contrast imaging, researchers continue to grapple with an inherent trade-off between resolution and sensitivity. When you optimize for higher resolution, you’ll often notice decreased sensitivity in detecting subtle contrast variations within biological specimens.
- Resolution diminishes when you reduce the condenser iris diaphragm size
- Halo and shade-off artifacts can obscure fine cellular details
- Light scattering in thick specimens compromises sensitivity
- Higher resolution settings may fail to detect low-contrast features
- Enhancing either resolution or sensitivity typically comes at the expense of the other
This balancing act presents a particular challenge when you’re examining transparent biological structures where both fine detail and subtle contrast differences are essential for accurate analysis and interpretation of cellular components.
Computational Resource Requirements
As digital phase contrast imaging techniques grow more sophisticated, you’ll encounter significant computational demands that can limit practical applications in both research and clinical settings.
Processing phase contrast images requires substantial hardware resources, with high-speed processors and ample RAM becoming essential rather than optional.
When you’re analyzing high-content screening datasets, memory usage becomes a critical bottleneck, especially when implementing deep learning models that need extensive image banks for training.
High-resolution images (200 × 200 pixels or larger) further strain your computational resources, potentially slowing real-time analysis.
You’ll find that computational efficiency is particularly challenged when correcting for phase contrast artifacts like halo and shade-off effects.
These complexities necessitate optimization of both your hardware configuration and software algorithms to achieve timely, accurate results.
Reconstruction Algorithm Limitations
When implementing phase contrast image reconstruction algorithms, you’ll encounter several fundamental limitations that can compromise analysis accuracy. Current approaches struggle with artifacts and optical variations that obscure critical cellular details.
- Halo and shade-off artifacts frequently mask important cellular structures, hindering segmentation efforts.
- High cell density specimens (particularly 3T3 cell lines) create challenging conditions for effective image processing.
- Inconsistent algorithm performance across cell lines (A549, Huh7, 3T3) as shown by varying MSE values.
- Incomplete modeling of phase contrast optics hampers development of more robust reconstruction methods.
- Regularized quadratic optimization functions don’t adequately account for specimen-specific characteristics.
These limitations are particularly pronounced when you’re working with specimens that exhibit low signal-to-noise ratios, making accurate pattern recognition difficult without specialized algorithmic adaptations.
Future Trends in Automated Phase Contrast Image Interpretation
The landscape of automated phase contrast image interpretation is rapidly evolving with revolutionary advances in artificial intelligence and computational techniques.
You’ll see machine learning algorithms, especially CNNs, dramatically improving cell counting accuracy and efficiency in the coming years. Automated phase contrast image analysis will increasingly leverage data augmentation to create robust models that perform well across diverse cell lines.
Watch for sophisticated restoration algorithms that eliminate halo and shade-off artifacts, greatly enhancing segmentation outcomes for live cell imaging.
The integration of multiple imaging modalities—combining phase contrast with fluorescence microscopy—will provide extensive cellular insights while minimizing photodamage.
Future developments will tackle persistent challenges like high cell density analysis and low signal-to-noise ratios, opening new possibilities for complex biological sample examination.
Frequently Asked Questions
What Is Digital Phase Contrast?
Digital phase contrast is a technique where you’ll use digital imaging to enhance microscopy images of transparent biological samples. It analyzes light phase shifts through specimens, allowing you to see living cells without staining them.
What Is a Phase Contrast Image?
A phase contrast image is what you’ll see when transparent specimens are made visible without staining. You’re observing differences in refractive index, where optically dense areas appear darker through specialized microscope components.
When Would You Use the Phase Contrast Microscope?
You’d use a phase contrast microscope when examining living cells or transparent specimens without staining. It’s perfect for observing bacteria, cell development, tissue cultures, and forensic materials while maintaining sample viability.
What Is the Phase Contrast Microscope Analysis?
Phase contrast microscope analysis is your method for examining transparent specimens without staining. You’ll measure how light waves change as they pass through cells, revealing detailed structures and cellular dynamics in living samples.
In Summary
You’ve seen how digital phase contrast imaging has transformed from analog techniques to sophisticated computational methods. As you integrate these tools into your research, you’ll discover enhanced visual capabilities and quantitative analysis options. Despite current limitations, you’re positioned to benefit from AI advancements and automation trends that will continue reshaping cellular visualization. Your adoption of these evolving technologies will enhance both qualitative observations and quantitative insights.
Leave a Reply